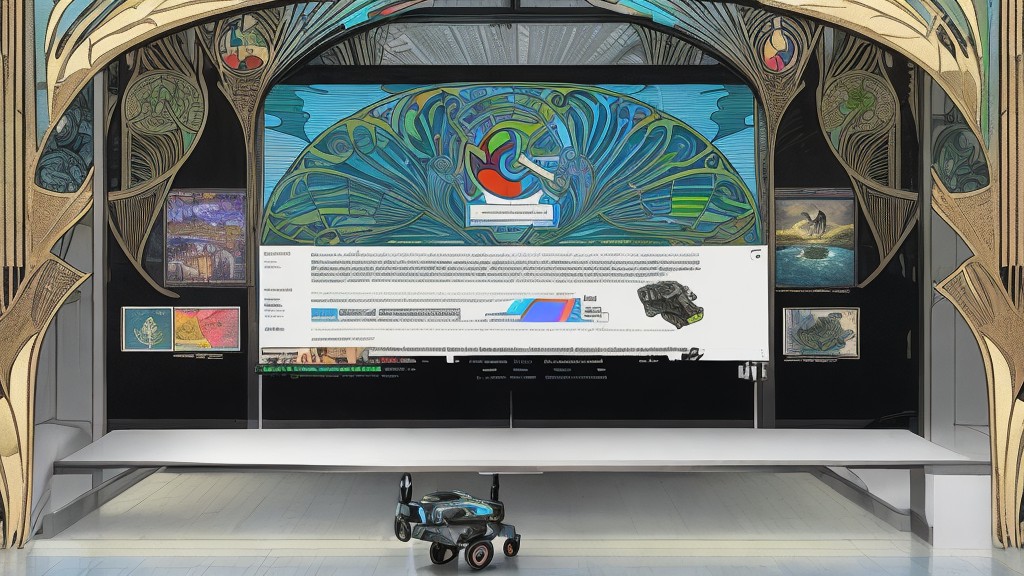
Google’s AI-Powered Search Generative Experience Revolutionizes Reading Online
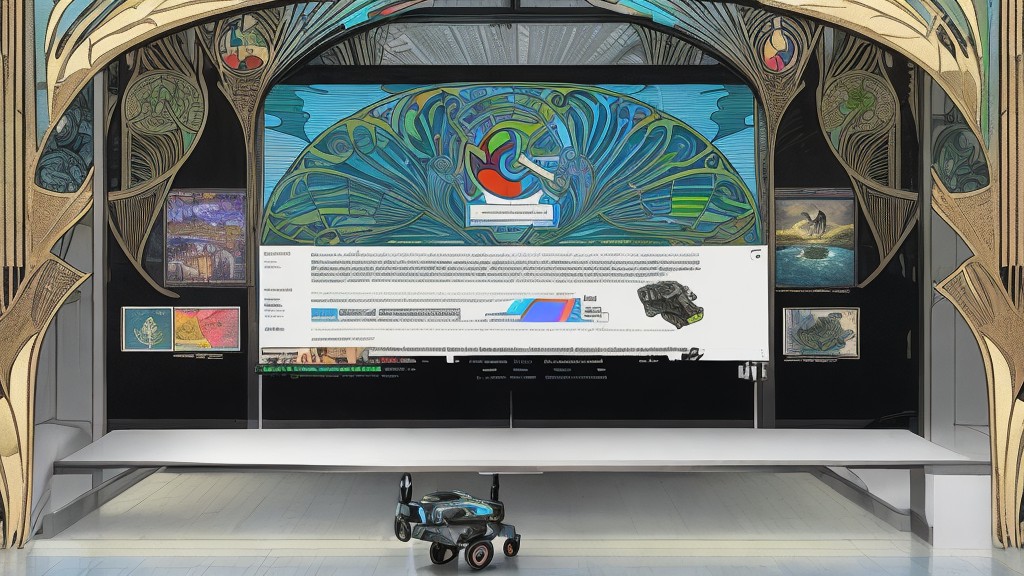
Google’s AI-Powered Search Generative Experience Revolutionizes Reading Online
Instant Summaries – A Time-Saver’s Dream
In the information age, with abundant content available at our fingertips, time is of the essence. Recognizing the desire for concise content digestion, Google has launched a novel feature within its Search Generative Experience (SGE) that promises to elevate your browsing experience. Imagine clicking on an in-depth article and being presented with a succinct summary instantly – that’s the prowess of Google’s latest innovation.
Highlights from Google’s Announcement:
In-line Definitions: The days of toggling between tabs to understand unfamiliar terms are over. Google’s SGE seamlessly integrates explanations right within the generated summaries, ensuring an uninterrupted reading flow.
Coding Made Simpler: For the developer community, SGE promises enhanced clarity. By offering crisp overviews of coding resources, it aids in fostering a more intuitive coding journey.
Empowering the Modern Learner: As online learning gains traction, the generative AI functionality within Google SGE steps in as a valuable ally. By summarizing intricate content, it aids learners in quickly grasping complex topics.
It’s clear this isn’t just about brevity. Google is taking bold steps to redefine how users interact with the vast expanse of online content. Their initiatives echo a singular goal: making the digital realm more user-centric.
For those eager to explore this transformative feature, Google is welcoming feedback and participants for its experimental phase. To dive into this enriched browsing experience, enthusiasts can sign up through Search Labs across multiple platforms, including Android, iOS, and Chrome desktop.
In conclusion, as we navigate an ever-growing web, tools like Google’s SGE are not just luxuries but essentials. They represent the next step in ensuring the internet remains a space of efficient knowledge exchange.