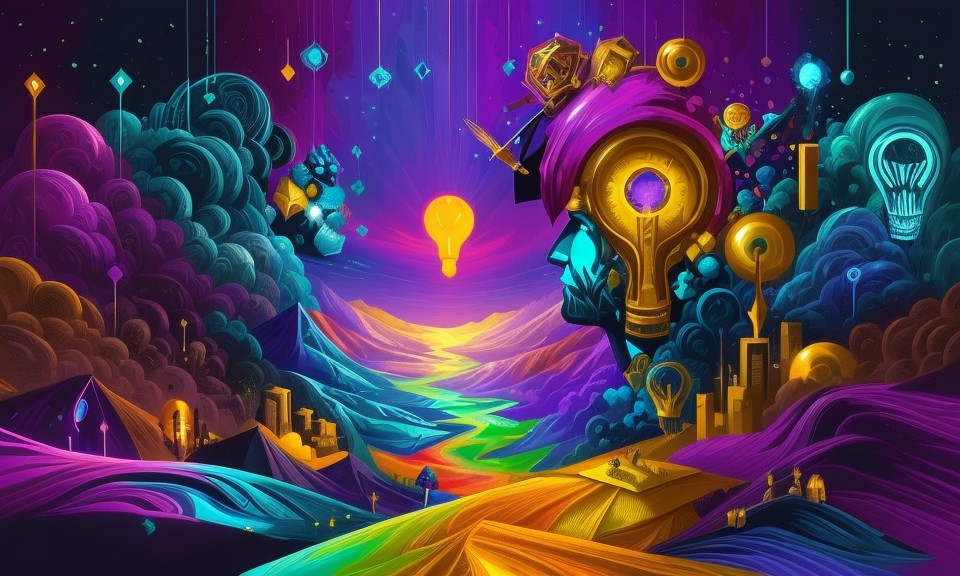
Visionary PhDs in LLMs – Forge the Future with RediMinds
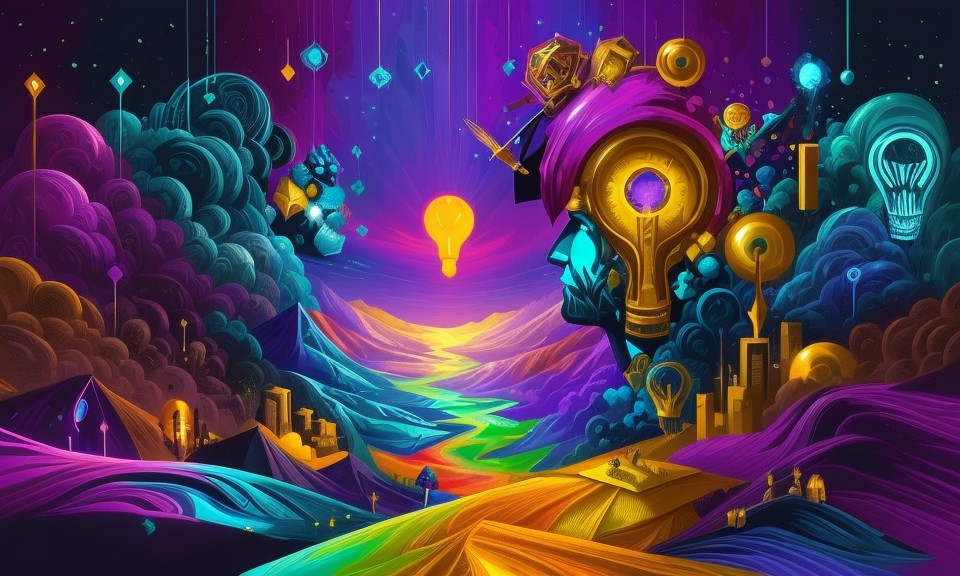
Visionary PhDs in LLMs – Forge the Future with RediMinds
In the swiftly evolving landscape of AI, staying ahead isn’t just an advantage—it’s a necessity. At RediMinds, we’re pioneering the frontier of Large Language Models (LLMs), and our eyes are set on the horizon, seeking those extraordinary PhD minds deeply immersed in the realm of LLMs. We’re not just following the evolution; we’re leading it.
Anticipate, Innovate, Lead:
Are you a PhD holder whose pulse races at the thought of LLMs? Do you find yourself not just navigating but foreseeing the future of AI architectures? If the answer is a resounding “Yes,” then you’re the visionary we seek. RediMinds is calling for brilliant, entrepreneurial PhDs ready to dive deep into the specifics and niches of LLMs—to become the unparalleled experts of tomorrow.
Your Expertise, Our Platform:
Imagine a place where your deep domain knowledge meets the resources to revolutionize LLMs. A space where your insights into the next big architectural leap in AI are not just welcomed but celebrated. At RediMinds, your foresight into what’s coming in LLMs isn’t just an asset; it’s our biggest priority.
Why RediMinds?
- Lead with Insight: Spearhead projects that redefine the boundaries of LLMs.
- Empower Innovation: With unique resources at your disposal, bring your visionary ideas to life.
- Shape the Future: Use your expertise to contribute to AI solutions that address real-world challenges, from enhancing knowledge access to solving complex societal issues.
Join the Revolution:
We’re on a quest for those who not only know the current landscape of LLMs but have the foresight to predict and shape its evolution. It’s about being proactive, not reactive. It’s about setting the trends, not following them.
Are you ready to be at the forefront of the next big breakthrough in LLMs? RediMinds offers you the stage to turn your visionary insights into impactful realities. Email us at talent@rediminds.com and let’s initiate an exciting conversation.
Let’s Create Magic Together:
Dive into the world of RediMinds, where your pioneering spirit in LLMs finds its true calling. Join us in our journey to transform the future, one innovative solution at a time. The realm of AI awaits your expertise. Let’s forge the future together.