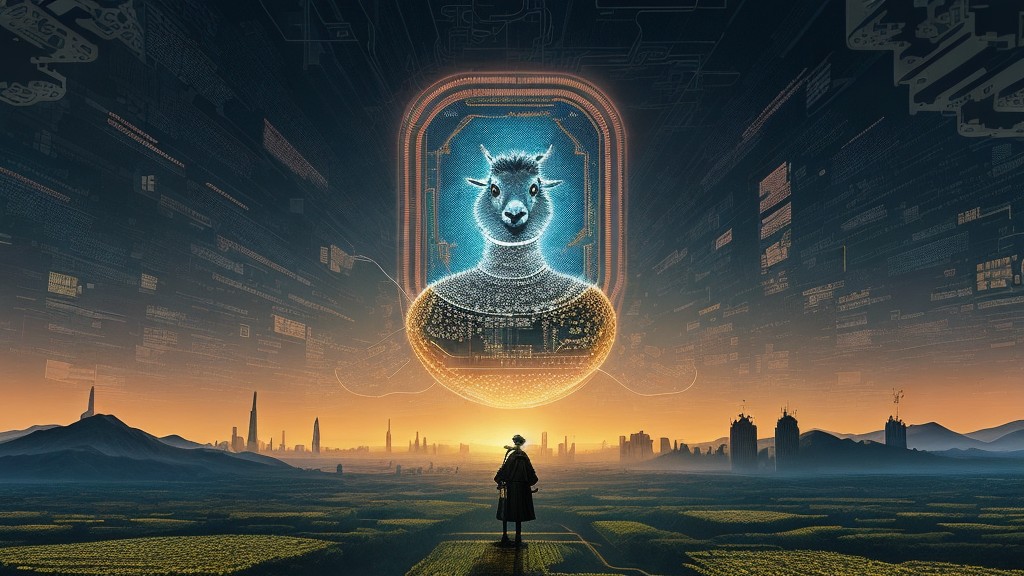
Direct Preference Optimization: A Paradigm Shift in LLM Refinement
The AI realm is witnessing yet another transformative development with the introduction of the Direct Preference Optimization (DPO) method, now featured prominently in the TRL library. As the journey of refining Large Language Models (LLMs) like GPT-4 and Claude has evolved, so too have the methodologies underpinning it.
Historically, Reinforcement Learning from Human Feedback (RLHF) stood as the foundational technique in the last stage of training LLMs. The objective was multifaceted: ensuring the output mirrored human expectations in terms of chattiness, safety features, and beyond. However, integrating the intricacies of Reinforcement Learning (RL) into Natural Language Processing (NLP) presented a slew of challenges. Designing an optimal reward function, empowering the model to discern the value of states, and averting the generation of jargon and gibberish all formed part of a delicate equilibrium.
This is where Direct Preference Optimization (DPO) comes into play. Marking a departure from the conventional RL-based objective, DPO provides a more direct and lucid objective, primarily optimized using binary cross-entropy loss. The overarching implication? An LLM refinement process that is considerably more straightforward and intuitive.
Delving deeper, an insightful blog post illuminates the practical implementation of DPO. The article delineates the process by which the avant-garde Llama v2 7B-parameter model underwent fine-tuning via DPO, leveraging the Stack Exchange preference dataset. This dataset, replete with ranked answers sourced from an extensive range of Stack Exchange platforms, serves as a rich resource for the endeavor.
To encapsulate, this development signifies a pivotal moment in the evolution of LLM refinement. The Direct Preference Optimization technique beckons a future that is not only streamlined and efficient but also transformative for the larger AI sphere.
Key Takeaways:
- The transition from RLHF to DPO heralds a simpler era of LLM refinement.
- DPO’s optimization hinges directly on binary cross-entropy loss.
- The pioneering Llama v2 7B-parameter model underwent fine-tuning via DPO, drawing upon the invaluable Stack Exchange preference dataset.
Given the advent of Direct Preference Optimization, the future of AI appears even more boundless. As the landscape of LLM continues to evolve, DPO is poised to play an integral role in shaping its trajectory.
Open Dialogue:
The AI community thrives on collaboration and exchange. How do you envision DPO reshaping the LLM ecosystem? We invite you to share your insights, forecasts, and perspectives on this exciting development.