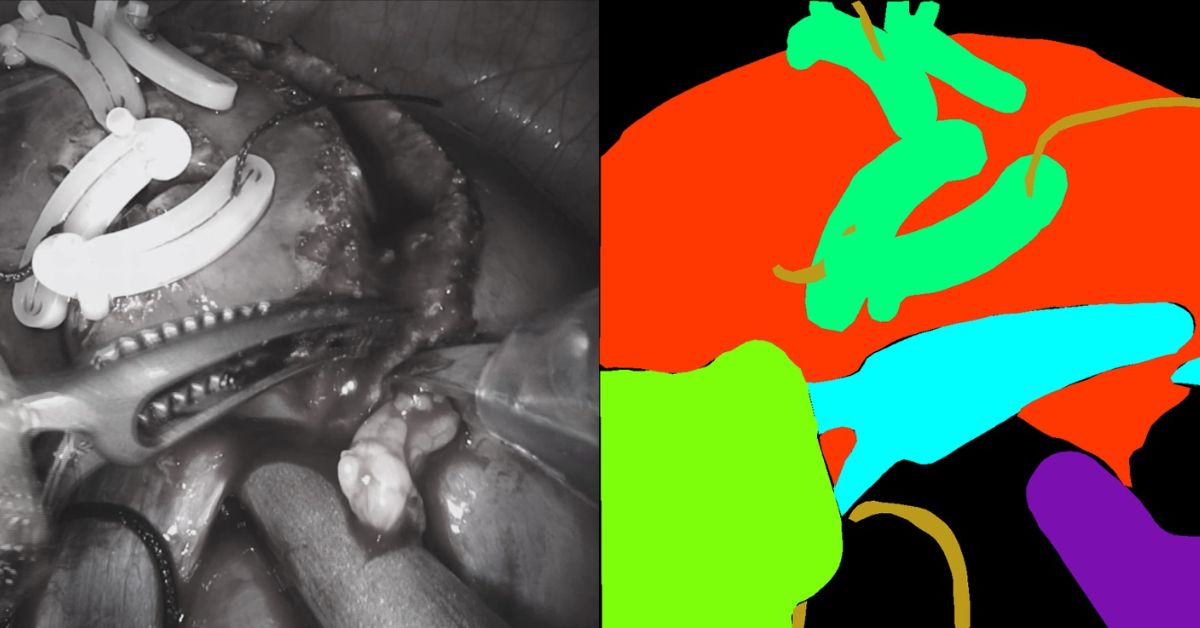
2D Semantic Segmentation for the Improvement of Minimally Invasive Surgery
AI-enabled surgical tools assisting in – or even taking over – highly complex surgeries could be on the horizon. But to make this a reality, the abilities of AI developers have to combine with the knowledge of medical professionals.
Let’s get into how 2D semantic segmentation is one way that professionals are collaborating and making huge strides in the right direction.
What is 2D Semantic Segmentation?
2D Semantic Segmentation is the process of associating every pixel of an image with a class or label – Check out this example of semantic segmentation of a road.
Now imagine this process being carried out on pictures from open surgery to determine labels in the images.
What is Minimally Invasive Surgery?
Minimally invasive surgery (MIS) is a great way to reduce recovery time and pain. MIS also allows surgeons to perform surgeries when more invasive surgeries are not the best option for a patient’s outcome.
Combining MIS and 2D Semantic Segmentation to Improve Surgical Accuracy
With robot-assisted surgery, the accuracy of the surgery nearly doubles. Surgeons’ accuracy has been greatly improved using articulated tools and high-fidelity 3D vision, which gives them more precision and control during operations.
This means there’s less risk of mistakes and complications – which is good news for surgeons and patients alike. But it can be challenging to locate the structure that needs to be operated on.
2D Semantic Segmentation helps with this difficulty by mapping out structures and providing accurate results.
An eventual objective for robotic surgery could be to perform surgery independently and with more precision than human surgeons. However, multiple technological components are required to achieve this goal.
2D Semantic Segmentation in Action
Despite the lack of large volumes of data, RediMinds took on the challenge of conducting semantic segmentation of surgical pictures into a set of medical equipment classes and anatomical classes.
The medical devices were classified as da Vinci instruments, drop-in ultrasonography probes, suturing needles, suturing thread, suction-irrigation devices, and surgical clips.
This collection included all non-biological items depicted in the photos.
The kidney parenchyma, renal fascia and perinephric fat, which we referred to as the “covered kidney,” and the small intestine were the anatomical classes. The rest of the anatomical items in the scene were assigned to a background class.
What’s Next for Robot-Assisted MIL?
Endoscopic vision integration with pre- and intra-operative medical imaging methods is the next revolution in enhancing surgeons’ skills.
To show this data carefully and intelligently, without cluttering the surgeon’s vision, one must first comprehend what objects are in view of the endoscope and what sections of the picture they represent.
This can help a surgeon better comprehend the anatomy they’re dealing with or the work they’re doing.
To accomplish this, the endoscopic camera’s pictures must be segmented pixel-by-pixel using deep convolutional neural networks (CNNs). Large volumes of labeled data are required to successfully train and assess these models, which are currently lacking in the medical sector.
As a result, performance improvements in general computer vision are not often seen in medicine, particularly surgery.
What’s Holding Back the Development of These Tools?
For artificial intelligence and ML model building, vast quantities of high-quality annotated surgical recordings and their metadata are required.
Current supervised learning approaches are not scalable because of the work that goes into the anatomical labeling of objects.
Also, it isn’t easy to correctly classify soft tissue in data without knowledge as a physician or surgeon meaning flaws in the labeling process could affect model accuracy.
The absence of incentives for surgeons to provide the high-quality surgical data required is a significant impediment to developing these tools. The model accuracy attained is not accurate enough for this type of technology to be used on humans, but there is potential for this in the future.
Robot-assisted MIS has changed patient care, offering benefits such as a decrease in injury, quicker healing, and an expanded treatment population. But the next step is the creation of AI tools that can perform surgery precisely and independently.
The first step to this creation is the ability for models to segment a surgical scene accurately.
Right now, there are many challenges to be faced when trying to complete this task.
But when these challenges are solved, many practical applications will be available for the training of surgeons and the development of AI safety tools in combination with augmented reality and virtual reality.