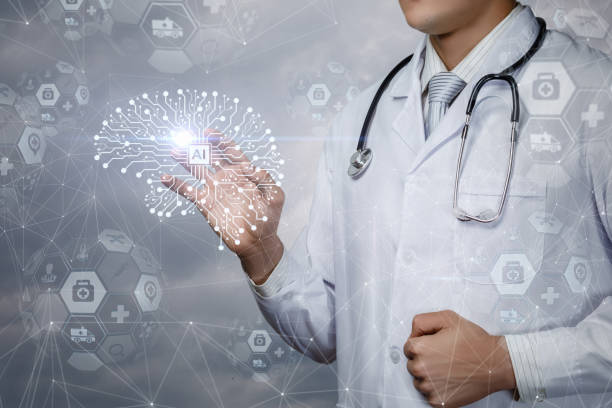
AI in Healthcare: 6 Applications of AI in Medicine
by Madhu Reddiboina | May 23, 2022 | Health Care
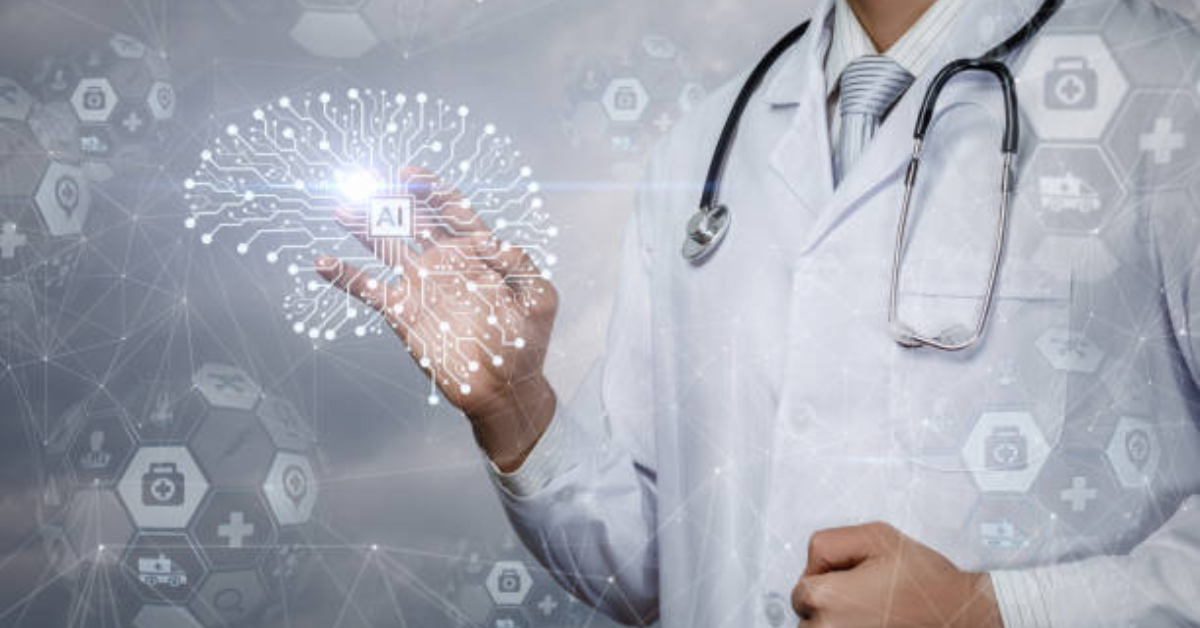
6 Exciting AI Applications in Healthcare
But AI isn’t the wild, human-replacing technology we see in movies like The Matrix.
Rather than replacing humans with sentient robots, AI is working to provide us with the knowledge and tools we need to revolutionize the way we live and work – and healthcare is a hotspot for AI development.
Let’s explore what AI means for healthcare and the exciting use cases making a difference in patient care:
- What is AI in Healthcare?
- Types of AI in Healthcare
- Why Does AI in Healthcare Matter?
- Where is AI Used in Healthcare? The 6 Exciting Applications
- Ethical Challenges of AI in Healthcare
- Final Thoughts
What is AI in Healthcare?
With the strong desire to become more accurate, efficient, and user-friendly present in all clinical settings, AI is a powerful tool to achieve these goals.
Nearly 80 AI algorithms are already approved by the Food and Drug Administration (FDA).
And this is only the beginning of the many exciting applications of AI in healthcare. With the power to augment our understanding, there is no limit to what we can do with advanced technologies in medicine.
Before we discuss some use cases of AI, what types of AI are contributing to these advances in healthcare?
Types of AI in Healthcare
Machine Learning in Healthcare
Machine learning algorithms that improve over time are capable of more accurately predicting healthcare outcomes. By processing huge volumes of data, ML technology can assist medical professionals in decision making, identifying diseases, and developing treatment plans.
A key use of machine learning technologies in healthcare is to predict what treatments are likely to be successful for a given patient, based on the context given.
Natural Language Processing
Natural language processing (NLP) is the branch of AI concerned with processing and manipulating spoken or written data that has been generated by humans.
The potential applications of NLP are endless, with this technology currently being used to recognize voice commands, organize written clinical data, and respond directly to patients via chatbots. But more on that later.
Robotic Process Automation
Robotic Process Automation (RPA) is the automated technology that can remove menial or repetitive tasks from the hands of professionals – a fantastic opportunity for healthcare organizations to increase efficiency, reduce costs, and ultimately provide better care to their patients in the process.
Use cases of RPA can include anything from basic admin tasks to processing billing and online communications.
Medical Robots
Physical robots have already been assisting with the provision of healthcare for some time. They’re already assisting with surgery, becoming prosthetics, cleaning, and assisting in pharmaceutical storerooms to retrieve the correct drugs.
These are just 4 key technologies that are being used in healthcare right now, but why does it matter that we keep moving towards AI-driven healthcare technology?
Why Does AI in Healthcare Matter?
How would you like to have your surgeon gain confidence through intelligent computer models that provide more clarity on your individual anatomy?
Or have an algorithm use your previous medical history to predict your likelihood of different adverse outcomes with greater certainty?
That’s exactly what some of the novel developments of AI in healthcare are achieving. Not only are AI technologies assisting in surgery and organizing medical data, they’re making health conditions and complications more predictable to prevent serious outcomes with the earliest warning signs.
Where is AI Used in Healthcare? The 6 Exciting Applications
Healthcare has been at the forefront of AI development for a number of years. Here are 6 areas in which AI is already transforming healthcare:
- Storing Electronic Health Data
- Automatic Image Analysis
- Predictive Analytics
- AI Chatbots
- Surgical Robots
- Medicine and Pregnancy
Storing Electronic Health Data
Over 80% of hospitals and physician practices are now using Electronic Health Records (EHR), which have revolutionized medical documentation and collaboration.
With the ability of EHRs to digitally store countless pieces of patient medical data, is the need for a system to sift through all of that information – enter AI technology.
With the help of AI, important information can be automatically pulled from countless types of clinical notes, diagnoses, order entry, and billing to be simultaneously placed into the hands of medical providers.
In 2018, Amazon launched their Comprehend Medical system based on natural language processing (NLP) and machine learning (ML). Their NLP model is trained to read medical documents and audio transcripts, while the ML algorithm extracts the relevant information and transforms it into an easy-to-read format for physicians.
Not only that, AI software can work alongside physicians and patients to transcribe conversations in real-time and automatically upload information to the appropriate section of a patient’s EHR. Some programs like DeepScribe’s AI average less than one edit per note – hugely reducing time spent on documentation and improving accuracy.
Cloud-based EHRs also allow that same patient data to be shared securely and quickly between medical providers or public health agencies with minimal error.
Throughout the COVID-19 pandemic, burnout among medical professionals gained global attention and efforts are being made to address the problem that has been occurring for years with technology that makes some efforts to reduce their heavy workload.
Time is of the essence in medicine, and AI allows the allocation of time towards tasks of the greatest priority.
AI can even be used to draw information from wearables into the EHR. Studies have highlighted the benefits of using technological vital systems such as electronic blood pressure cuffs, Apple Watches, and Fitbits to automatically upload a patient’s vitals into their EHR.
Physicians are even leveraging the AI technology in wearables for the detection of atrial fibrillation and other diseases by monitoring cardiovascular data.
Automatic Image Analysis
72.4% of AI-based FDA-approved medical devices and algorithms are innovations in radiology.
It can be difficult to detect adverse outcomes on imaging and scans and an immense amount of specialized training is required to do it correctly. The timeline for diagnosis and intervention relies upon the ability of medical professionals to quickly and accurately read the images.
Through structure recognition training, AI technology can help radiologists identify difficult-to-detect fractures that may otherwise have gone undetected by alerting them to structures, abnormalities, or details that may not be visible at first glance.
Hip fractures can sometimes be particularly challenging. Over 300,000 people over 65 are hospitalized every year in the US for hip fractures diagnosed using x-rays. But when the fracture is hidden under soft tissue, it can be difficult for radiologists to detect and treat it.
A study published in the European Journal of Radiology describes the use of Deep Convolutional Neural Networks (DCNN) to help radiologists identify hip fractures.
With the help of this technology, Radiologists reading hip x-rays were able to spot 88% of fractures, versus 83% without.
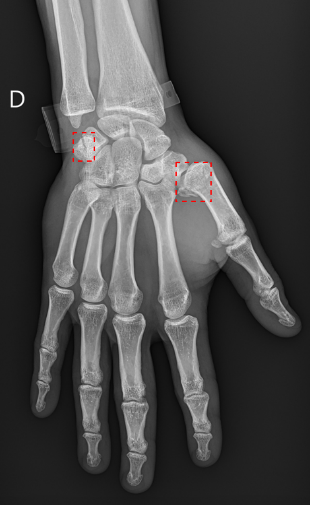
Ultimately, getting more patients the treatment they need faster.
Predictive Analytics
Treating disease and providing care requires doctors and patients to make decisions based on the information they have available and their understanding of the risks at play.
Limited information, hard-to-detect diseases, and rare complications can make it hard to plan medical treatment without the help of technology that can help predict outcomes.
Predicting Illness
When conditions and complications become more predictable, better care can be provided. With the help of AI, rare and hard-to-detect conditions become more detectable, giving professionals a better understanding of their patient’s needs and the opportunity to provide more targeted screening or care.
In diseases like ovarian cancer, early detection is essential, but screening methods are limited. With the help of AI, novel tests like microRNA (miRNA) screening are being developed and early research shows nearly 100% accuracy in identifying ovarian cancer cases when combining miRNA screening and deep neural networks (DNN).
This research may well lead to simple blood screens on seemingly healthy women that will alert physicians to pre-symptomatic tumors so they can begin treatment earlier than ever before.
AI can also be used to predict complications or health problems just from electronic health records.
Once a patient begins care at a hospital, countless pieces of information are collected from check-in, follow-ups, tests, surgeries, medications, and more. Where existing risk measures like the Early Warning Score only take a few key variables from EHRs into account, AI can draw from the nearly 10,000 types of data for a single patient, hugely improving the predictive values of models.
For example, our team at RediMinds researched the application of natural language processing (NLP) in the ICU, using existing EHRs to more accurately predict the future.
Based on the first 24 hours in the ICU, our machine learning models can analyze the risk of in-hospital mortality or extended hospitalizations, alerting staff to at-risk patients early.
Predicting Transplant Waitlist Mortality
RediMinds has also made significant progress in predicting mortality among those on the liver transplant waitlist with cutting-edge AI models.
With around 15,000 Americans waiting for a liver donation every year, doctors are tasked with allocating the limited supply of organs for transplant based on two factors:
- Those most at risk of dying while waiting
- Those who have the greatest chance of successful transplantation
The Organ Procurement and Transplantation Network (OPTN) has used models like MELD-Na to begin allocation in hopes of minimizing wait time for the patients deemed most urgent.
But in recent years, there’s been concern about the effectiveness and fairness of these models.
In fact, women are more likely than men to die while waiting for a donor organ if this model is used for triaging patients.
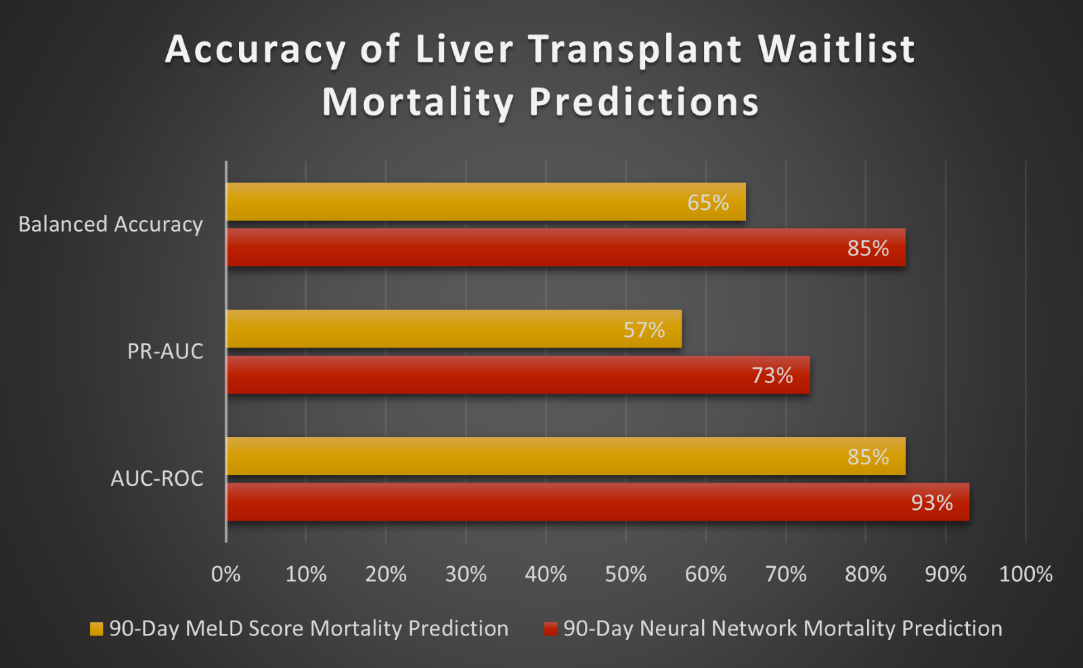
That’s why, to combat these disparities, RediMinds developed a 90-day mortality prediction model using pre-transplant information from patients registered with OPTN. The model is cross-validated with different age groups, ethnicities, and other factors to limit bias and make results more reliable and fair for all patients.
So far, RediMinds’ model has outperformed the MELD score in its predictions.
Clearly, given the disparities seen in existing models, it is essential to continue designing tools and building data sets that seriously consider and reverse inequities.
AI Chatbots in Healthcare
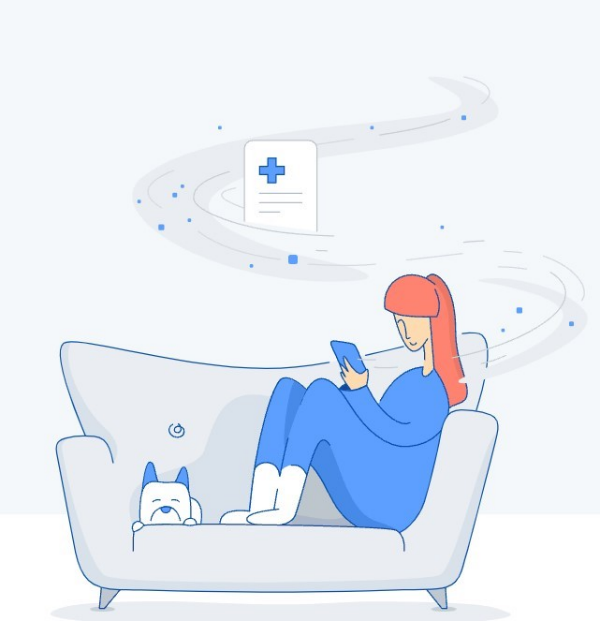
Chatbots have become the foundation of this online support.
They are highly trained computer programs or smart algorithms that pick up auditory or textual cues through conversations using natural language processing.
Some programs like Northwell’s Colonoscopy Health Chat are already reducing no-shows by providing educational resources in a responsive, conversational way to help ease patients’ concerns.
Other chatbots like Florence remind patients to take their medications through Facebook Messenger, Skype, or Kik. After inputting your medication, dosage, and frequency, Florence will message you when you need to take the pill. With patient input, Florence can also monitor body weight, mood, or menstrual cycle.
In the future, chatbots like Ada Health are expected to become the first point of contact for primary care. Answering basic questions, documenting complaints or concerns, offering solutions for simple medical issues, and managing appointments to free up time for medical providers to spend with the patients who need it.
These convenient pocket medical providers can also actually help increase people’s willingness to monitor their health by making it so easy, enabling greater predictive and proactive care in the long run.
The possibilities for chatbots in healthcare are endless.
Surgical Robots in Healthcare
AI has played a major role in the intelligence and automation of medical robots. Beyond simpler tasks like taking a patient’s vitals or reading clinical notes, there has been a shift towards developing robots for surgical procedures that require less invasive, more patient-tailored methods.
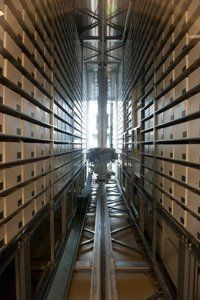
Other companies like Medineering are developing innovative surgical tools to increase patient comfort and limit the potential consequences of procedures.
They’ve developed flexible robots to be guided to the exact spot a doctor needs during an endoscopy. Instead of the tremor that naturally comes with human hands, these robots hold cameras completely still and can even deploy tools for anything from biopsies to wound cauterization.
Another prime example is the MRI and Ultrasound Robotic Assisted Biopsy (MURAB) project, aiming to make early cancer diagnoses easier and less invasive. Surgeons can steer the robot to a site of interest and use a novel MRI/ultrasound combination technique to create a 3D image of the area to be biopsied.
Surgeons can then handpick where they want to get a biopsy from and leave an incision the size of a paper cut as they retract the robot through the same opening. Limiting unnecessary navigation and cutting to decrease the risk of infections and make for a much easier recovery for patients.
Medicine and Pregnancy
When evaluating certain conditions, medications, or outcomes, it can sometimes become difficult to create prospective studies without breaking ethical guidelines.
Randomized controlled trials are widely preferred to determine causality and are commonly used to look at the side effects of medications. But some randomized controlled trials raise concerns about ethicality, safety, and feasibility.
For example, pregnant and postpartum—specifically lactating—women are one of the groups that have been systematically excluded from many areas of research due to the risk of unknown impacts on the pregnancy and child.
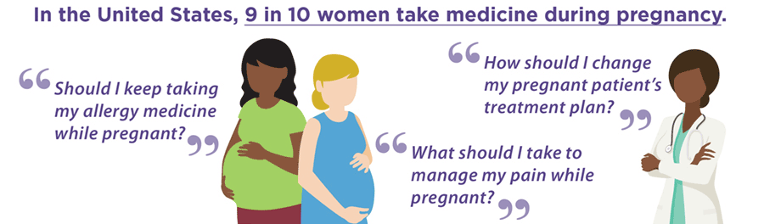
Over 50% of pregnant women take at least one prescription drug at some point in their pregnancy.
Without randomized controlled trials to collect the type of data essential to ensuring that medication is safe for women and their children, another method of determining safety is crucial.
That’s where retrospective analysis comes in – using AI to evaluate past data without putting participants in any future danger or medical uncertainty.
AI methods to analyze electronic health records can aid in the lack of knowledge about pregnancy and pharmaceutical consequences, ultimately helping pregnant women and their doctors make more informed medical decisions.
Instead of randomized controlled trials, a retrospective design can be used to look at what has happened in the past and why. Public registries have made this easier by providing longitudinal data of complex cases that would be time-consuming to compile retrospectively.
Ethical Challenges of AI in Healthcare
With any model mimicking human thoughts and behaviors, we must always pay extra attention to ethics.
When training models that determine important, high-priority details to predict outcomes and potentially save lives, it is crucial that focus is paid to the quality of data used in training.
Entered data should be accurate, unbiased, and not reflect the disparities we unfortunately still see in the medical field. Entering biased data into AI models will only create prejudiced outputs, which perpetuates the racism-based imbalance of power between patients and providers.
This prejudice is exactly what we are trying to prevent by creating these models in the first place.
Final Thoughts
AI and ML are not necessarily meant to replace human tasks, threaten our job security, or threaten our purpose. Instead, they can be used to reimagine what is possible and make work previously conducted solely by humans easier, more accurate, and more equitable.
AI-driven advances are freeing up more time for medical professionals and researchers to focus on tasks that need human intervention and driving more informed medical decisions that have a huge impact on improving the outcomes for patients.
Researchers across the globe are constantly working on new and exciting applications of AI in healthcare, progressing the field of medicine every day and ultimately helping to provide better care to patients.
As AI technology continues to expand into new realms of medicine, there are no limits to its potential benefits.