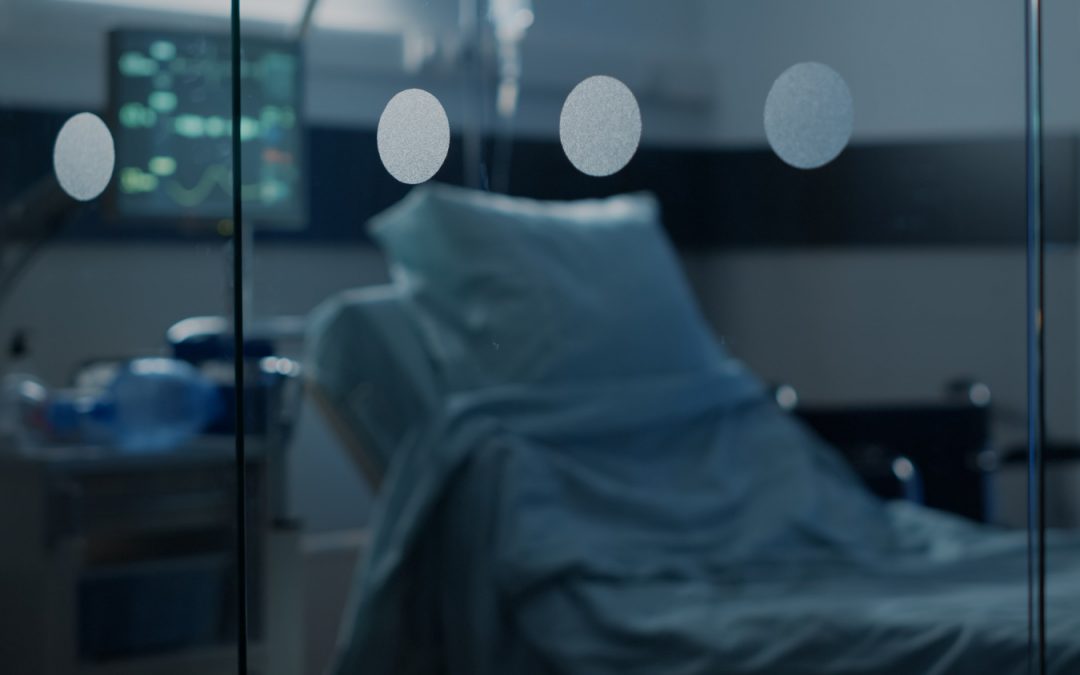
AI in ICU using Natural Language Processing on EHR
by Madhu Reddiboina | Aug 26, 2021 | Health Care
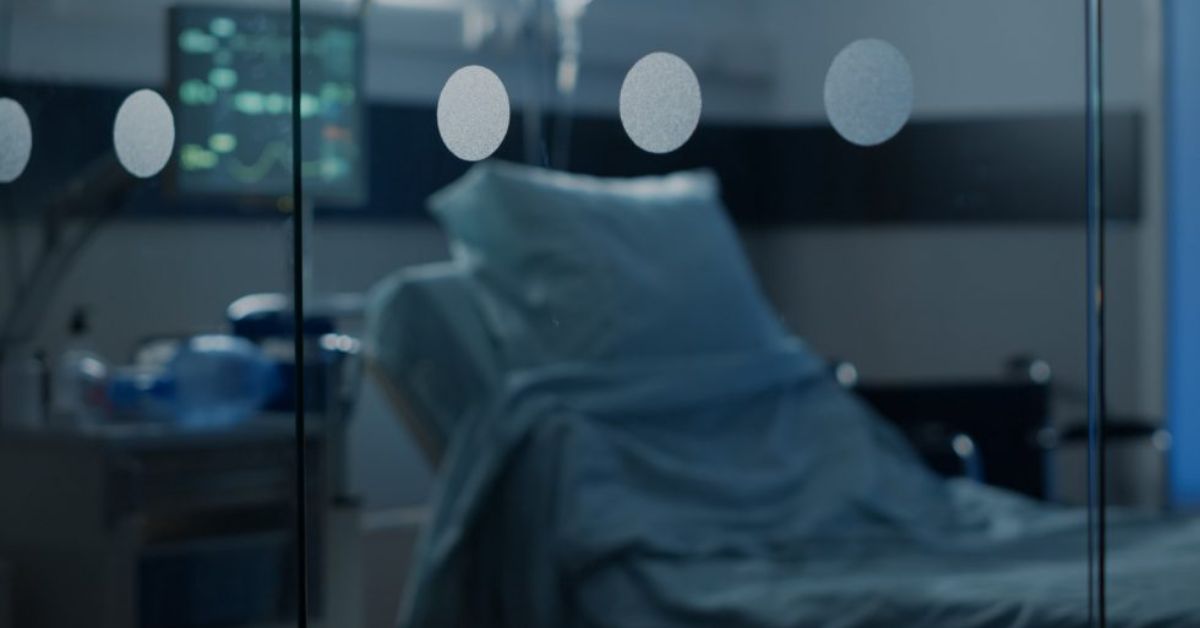
AI in ICU using Natural Language Processing on EHR
However, patient EHRs (Electronic Health Records) contain a large amount of information for every patient. In an ICU, around 9,000 to 10,000 different types of data can be collected for each patient. This poses a challenge for the development of AI and ML tools, which we will discuss further in this article.
The Challenge of ICU data for ML models
AI and machine learning (ML) tools models are the future of medical care. These tools allow hospitals and medical facilities to provide better care for patients and research new surgical techniques and medications. However, researching how well tools and models work in the medical environment and training ML models with past data is a vital first step.
The best way to analyze data and perform research like this is by analyzing the data of past patients. As stated earlier, EHRs have tons of patient data, so much that it’s complicated to train a ML model using this data. There are many elements and information collected on patients in the ICU. From the moment they arrive at the hospital, to follow-ups, to surgeries, there are so many pieces of information. This information isn’t even fully structured data. Most of it’s just doctors’ notes, random information, and pictures, and it’s all in different formats.
Training a ML model is a huge challenge when trying to analyze historical data from the ICU. To train models, the data has to be formatted in a specific way. For instance, one doctor at one hospital may label something as one variable, while another doctor may label it as another variable. The variables are usually the same, although the names differ amongst EMRs.
In certain EMRs, Heart Rate may be coded as ‘Heart Rate’, whereas in others it is coded as ‘HR’. This does not prevent model training, but it makes transferring a model from one facility to another difficult. To accomplish the model transfer in this situation, it would be necessary to map new data variables to old data variables names, then conduct data processing and model training processes. Trying to apply a learned model or procedure to several additional hospitals increases the complexity. Hence why interoperability standards like HLv7 and FHIR are created to make data storage more standardized.
Now, multiply that situation by thousands, and now there is a vast amount of data that needs to be cleaned before researchers can use it to train a ML model. Data cleaning is a huge process for data scientists. Researchers spend a lot of their effort gathering and preparing chaotic digital data before using it to build algorithms. Data comes in all different forms. Data scientists attempt to automate as many procedures as possible, but human language is ambiguous and requires data to be cleaned manually.
In fact, some research has indicated that preparing and maintaining data for analysis takes up around 80% of a data scientist’s work. For ICU data, this would mean going through thousands of variables to ensure they are all formatted correctly. However, it’s vital to have clean data because it provides better outcomes and more accurate ML models.
Natural Language Processing as a Solution
A potential solution to this issue that RediMinds have researched is the application of natural language processing (NLP). NLP analyzes language in text and speech using computer-based approaches. Think of it like this. When we talk to Google Home or Alexa, those devices break down our sentences into tokens. For instance, if you say, “Alexa, light switch on,” the device breaks down the sentence to “light,” “switch,” and “on” and performs an action that it has been trained to do based on these tokens.
Regarding using NLP in the ICU for historical data analysis, NLP models can look for words in EHR notes most commonly used in patients with certain conditions. For example, a patient with a heart issue probably has several words like “cardiac” or “arteries” in their notes. These words can be collected by a ML model, which then can train models on what happens next to this patient.
RediMinds investigated this technique because handpicking or curation of variables takes a long time and may result in the omission of potentially significant data items. The RediMinds team was able to analyze and use data for model training without rejecting large quantities of material by utilizing NLP methods.
Wrapping Up – Why is this Important?
Using NLP techniques to process both structured (tabular data) and unstructured (Physician’s Notes) cuts down on the time it takes to develop new models for patients. This technique also makes it easier to develop subsequent models because researchers can use the same data. Finally, this technique leverages entire datasets instead of hand-selected variables, which helps eliminate biases of what researchers think is important for the model. With this technique, researchers can tap into valuable insights that may have gone previously unknown due to hand-selecting variables to include in models.
Using NLP also leads to interoperability. With so many different ways to record data, it is nearly impossible to build ML models to make ICU predictions efficiently. However, this technique can help with gathering clean data for building future models.
Based on the first 24 hours in the ICU, ML models can answer questions about patient survival chances, how long patients will be hospitalized, and make several other predictions. If a ML model predicts that a patient will not survive more than a certain amount of hours, then this indicates to doctors and ICU staff that this patient needs to be checked on frequently. This type of AI tool in the ICU has the potential to save lives.
To learn more, check out the case study.