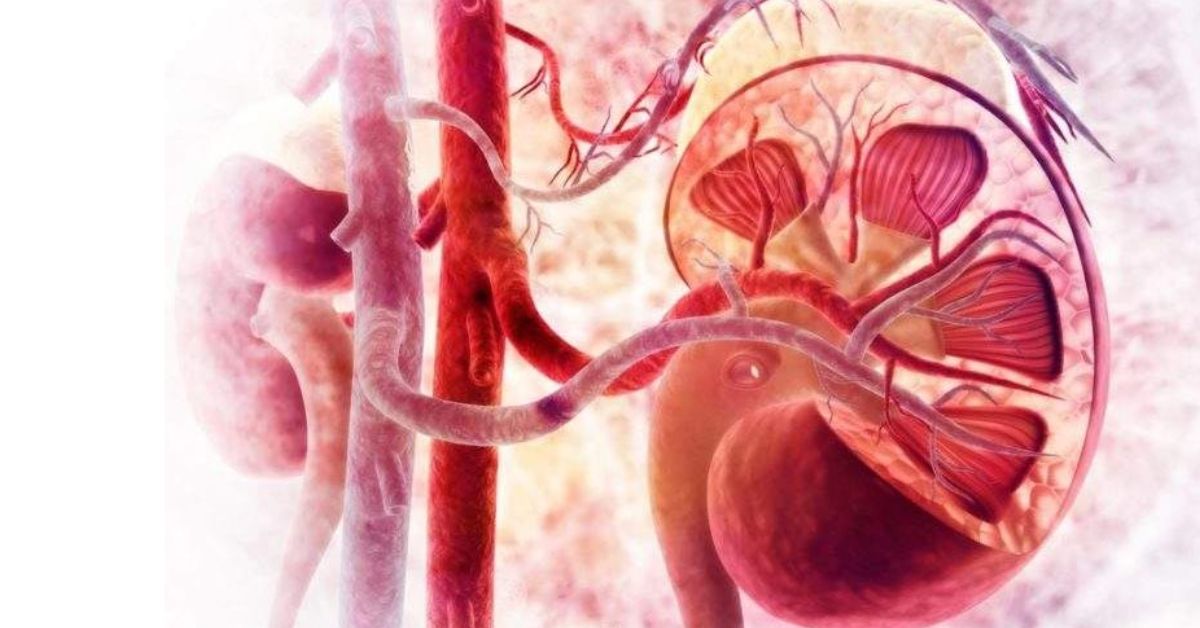
Use Machine Learning to Predict Acute Kidney Injury (AKI) and its sequalae
Problems with Conventional Prediction Models
In conventional approaches, the prediction models used for AKI rates were unable to be accurately applied to larger groups of patients as they ignore vast physiological time-series data. These limitations prevented them from being of any significant clinical value who are still spending a lot of time and resources trying to treat patients with post-surgical AKI.
How Machine Learning Improves AKI Prediction
The previously mentioned study included a group of 2,911 adults who had surgery at the University of Florida Health sometimes from 2000 to 2010. By using a combination of statistical analysis and machine learning algorithms, the researchers were able to create a perioperative model that was capable of attempting to predict the risk of these patients developing AKI during the course of their illness.
The results were segmented and analyzed as three separate time periods. The first was within three days after the date of the surgery, the second time period was within seven days of the surgery, and the third time period was from the date of the surgery to as far as the data went. One of the biggest differences in these models when compared to other existing ones was the inclusion of intraoperative physiological time-series variables.
By using this new prediction model, the researchers were able to achieve much higher accuracy when compared to the previous models across all three time periods. In total, the machine learning algorithm correctly reclassified 40 percent of patients who had originally received a false negative. These patients were now being classified as high-risk for developing AKI, which would have allowed them to get the preemptive treatment they needed.
When someone develops AKI, it makes them much more susceptible to developing future cases of AKI and also increases the likelihood of them developing morbidity, heart disease, a stroke, and much more. Therefore, the increased accuracy of this machine learning prediction algorithms means that significant amounts of money and man-hours will be saved trying to treat AKI and its related future health issues.
The results of this study indicate that with a machine learning-based prediction model, future predictions of AKI risk will soon be far more accurate in nation-wide predictions for any group of patients. To get more up-to-date news regarding AI and machine learning use in healthcare, make sure to follow our blog at RediMinds.