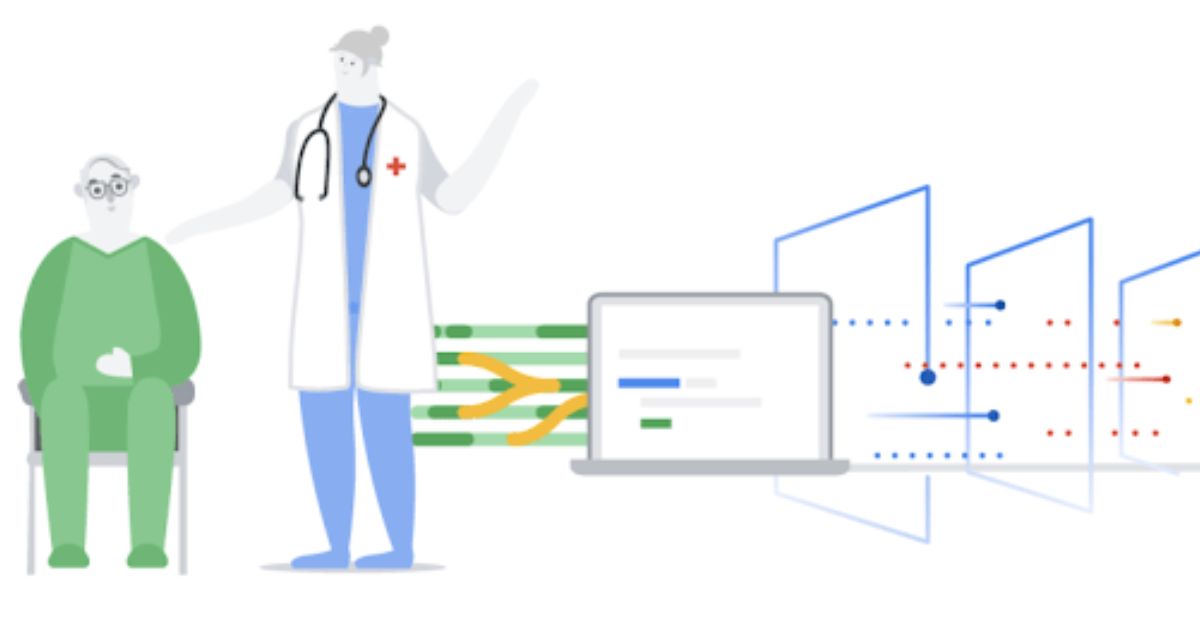
Google Taking on Healthcare and Clinical Artificial Intelligence
- In-hospital mortality
- Length of stay
- Readmissions
- And diagnosis at discharge.
They achieved all this using deep learning and fast healthcare interoperability resources (FHIR).
So what are EMR (Electronic Medical Records)?
Digital transformation of the healthcare industry is well underway. As part of that, EMR have allowed healthcare providers to document everything you’d find on a paper chart electronically, providing all the fundamental patient data medical staff need to make informed decisions about their patients.
Making patient records more accessible and theoretically more sharable between healthcare providers.
Which brings us to FHIR.
What is FHIR (Fast Healthcare Interoperability Resources)?
FHIR is the standard defining how healthcare records can be exchanged between different computer systems.
The aim is to allow developers to build standardized applications no matter how the original EMR data is stored, connecting healthcare providers to share data in a more effective way and removing the need to share patient data via PDF or paper copies.
Limitations of Traditional Machine Learning Models
Traditional machine learning and statistical models are built using a limited number of known variables from patient records ignoring most of the data that exists on every admitted patient.
Technically speaking, this work is very different.
They utilized all the patient data including encounters, labs, medications, notes, procedures, observations, and more to build deep learning models superior to work that predated it.
This paper entitled, Scalable and Accurate Deep Learning with Electronic Health Records published in NPJ Digital Medicine, is a masterpiece with amazing results in predicting patient outcomes using personalized predictive models.
The Study
In the study, the authors assumed the position that by representing a patient’s raw EHR data according to the FHIR format, deep learning can accurately predict outcomes and medical events regardless of the location of the patient from one setting to the next.
According to the authors:
“We validated our approach using de-identified EHR data from two US academic medical centers with 216,221 adult patients hospitalized for at least 24 h. In the sequential format we propose, this volume of EHR data unrolled into a total of 46,864,534,945 data points, including clinical notes.”
Events Predicted and Accuracy Rates Include the Following:
- In-hospital mortality – 93 to 94 percent accuracy
- 30-day unplanned readmission – 75 to 76 percent accuracy
- Prolonged length of stay – 85 to 86 percent accuracy
- All of a patient’s discharge diagnoses – 90 percent accuracy
- Impact of Deep Learning on Healthcare
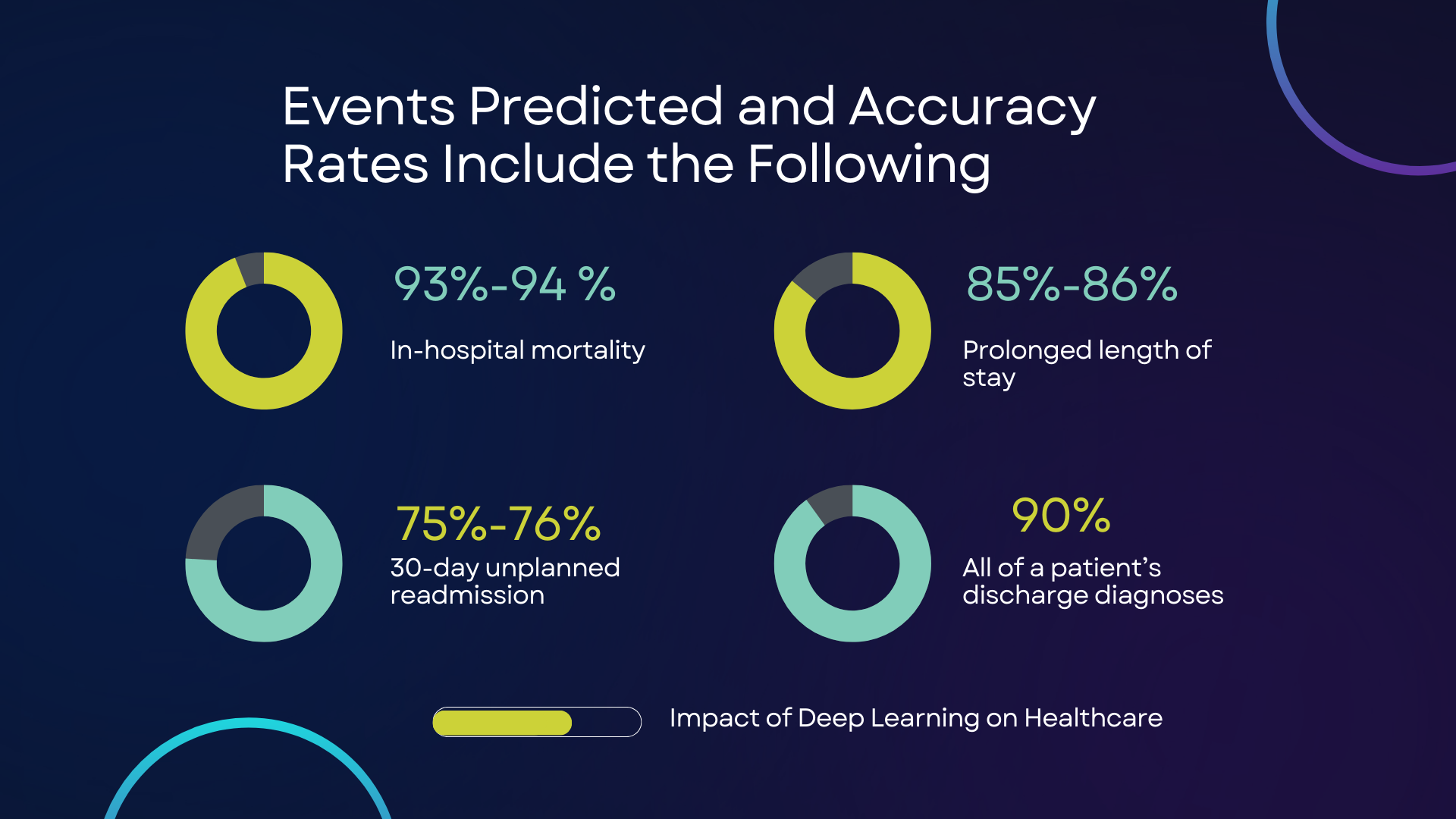
What This Means for Machine Learning in Healthcare
Deep learning techniques used to accurately predict medical events hold great potential.
Providing better care and monitoring of critical care patients while also optimizing hospital resources.
If put to work in a clinical setting, we can expect the machine and deep learning algorithms to improve the predictability of critical events and the care delivery workflow. Just like how AI algorithms are saving lives affected by hemodynamic instability.
Possibilities to use deep learning to improve the quality of care are endless – with the full benefits AI can have on the healthcare industry still yet to be discovered.
And healthcare isn’t the only area where AI is taking massive strides. Check out our article on how AI can make anyone a pro dancer.