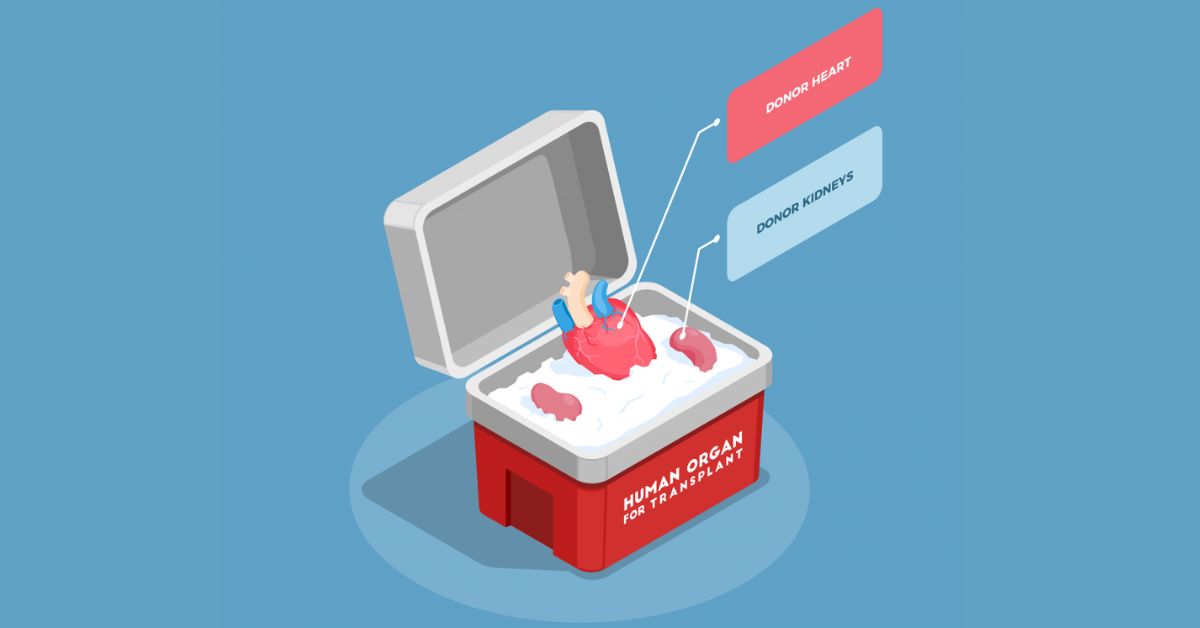
Using AI to Predict Mortality Risk of Liver Transplant Waitlisted Patients
The OPTN’s (Organ Procurement and Transplant Network) and United Network for Organ Sharing (UNOS) make up the transplant system in the US. OPTN/UNOS aims to allocate organs to candidates based on medical urgency while minimizing wait time and excluding patients for whom transplantation is unlikely to be successful. They do this by taking factors into account such as geography, organ size, blood type, and other medical factors. In 2002 OPTN and UNOS began using the MELD-Na (MELD-Sodium), formally Model for End-Stage Liver Disease (MELD), to allocate donor-livers to patients with the highest medical need.
The Issues with MELD
Patients on the waitlist who are judged to be at the highest risk of death are identified using current MELD and MELD-Na procedures. However, there is some question about whether this process is effectively and fairly identifying these patients.
For example, there are some gender-based disparities. Gender disparities in liver transplantation have been identified but are poorly understood. Women are 30% less likely than males to undergo liver transplantation, and this discrepancy has grown with the implementation of the MELD-based allocation system. Women are more likely than males to die while waiting for a donor organ in the MELD era.
This research discusses some of the gender disparities. The differences are most likely multifactorial and go beyond height and listing MELD score. One proposed factor of this disparity is a systematic bias in MELD score, which disadvantages women due to lower muscle mass and, hence, serum creatinine. However, no study could show that sex-adjustment of MELD score would eliminate this inequity.
Other factors like the differences in liver disease etiology and progression and the prevalence of hepatocellular carcinoma (HCC) have not been considered. Patients with HCC, typically males, get additional MELD points to help them get lung transplants when their biological score alone does not indicate the urgency of the need for surgery. However, it’s unclear if women’s increased risk of dying while waiting for a liver transplant is due to lack of access or biological factors.
Using Neural Networks to Predict Mortality
RediMinds used neural networks to determine if more effective models could be used alternatively to the MELD and MELD-Na methods to predict mortality rates of waitlisted patients. An artificial neural network is an effort to replicate the network of neurons that comprise the human brain in order for the computer to learn and make choices similarly to a human.
The data was obtained from UNOS, which comprised pre-transplant and post-transplant data on patients registered with OPTN. MELD score is a 90-day severity score for liver transplant patients, so using Neural Networks and a subset of the UNOS data, we used the performance of our 90 Day model against the MELD score for comparison.
After preprocessing data and building Neural Networks, for each outcome, model performance was assessed. The assessments used different subsets of data depending on ethnicity, gender, age, diagnosis, region, and year. To examine the difference in performance, the 90-day mortality prediction model was compared to the MELD score.
The models developed in this study worked well in predicted the mortality of waitlisted liver transplant patients. In fact, the 90-day mortality model significantly outperformed the MELD score in its predictions. The team also built models to estimate the probability of waitlist death at 180 days and 365 days. These other models also performed well.
Cross-validation is essential because it ensures the model is not biased, reliant, and can be used outside of one section or subset of data. To avoid the biases of MELD scores, the finished model is cross validated to check the performance of the model with different age groups, ethnicities, and various other factors.
Implications for the Future
These models can be used to identify individuals on the waitlist who have a high risk of mortality across different time periods and can be used as an alternative to the MELD score since it predicts outcomes with more accuracy. In addition, the methodology, techniques, and tools used to create these models can also be applied to many other surgical outcomes.
To learn more, check out this case study.
Appendix:
American Society of Transplant Surgeons (ASTS)
Organ Procurement and Transplant Network (OPTN)
United Network for Organ Sharing (UNOS)
Model for End-Stage Liver Disease (MELD)
MELD-Na (MELD-Sodium)
Hepatocellular carcinoma (HCC)
This isn’t the only way that our research into AI in healthcare is improving patient outcomes. Check out our guide to 6 exciting applications of AI in healthcare.