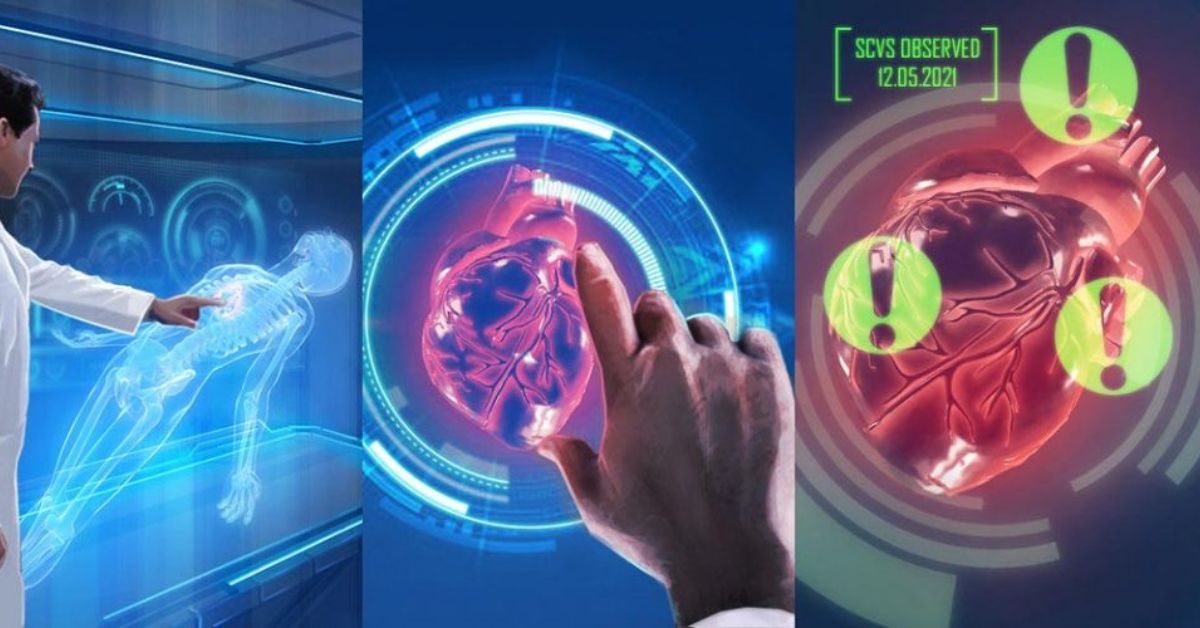
How AI based Clinical Decisioning Tools Can Lower Premature Deaths From Chronic Diseases
n a new study exploring AI predictions of premature patient deaths that was conducted by the University of Nottingham, a number of machine learning and cox regression models were compared in terms of their prediction performance.
Machine Learning Algorithms Outperform Traditional Approaches
Models developed took into account the demographics, biometrics, and lifestyle factors such as the dietary habits for each individual. After making the prediction, the results were tracked and directly compared against the 2016 death record information provided by other national sources. This allowed them to calculate how accurately the ML algorithms could predict premature deaths caused by chronic diseases. Deep learning model and Random Forest model out performed multivariate cox regression model which was focused on limited number of variables.
How AI Identifies the Risk of Chronic Diseases More Effectively
When compared against standard human-developed prediction models, which in this case was a Cox regression and a multivariate Cox model, the random forest and deep learning AI models were shown to be significantly more accurate. In fact, they were approximately 10 percent more accurate than the Cox regression and over 3 percent more accurate than the multivariate Cox model.
Even this slight increase in predictive accuracy can have significant impacts in care delivery throughout the United States and with active feedback loops, these models can be improved to reduce the 23 to 25 million premature deaths that the U.S. suffers every year.
By replacing the traditional approaches for model development with Deep Learning architectures and algorithms the next generation of clinical decisioning tools can be prospectively deployed into clinical settings. These more promising AI models can potentially detect and save millions of lives that are prematurely dying from chronic diseases. This mechanism can provide the clinicians an observation window wherein an adverse event is foreseen, and risk level assessed with an opportunity to administer appropriate preventative care and potentially avert the complications and premature death.
Through further testing, this team of healthcare data scientists will be able to determine just how successful this AI predictive model would be with other population groups. With enough progress, these models could soon be successfully used by doctors and other medical professionals in the treatment of chronic diseases as part of routine healthcare.Of course, the prediction of premature deaths in chronic disease patients is only one of many white space opportunities where AI can be used to improve clinical care. To get more industry news regarding the use of artificial intelligence in clinical care, make sure to subscribe to our blog at RediMinds.