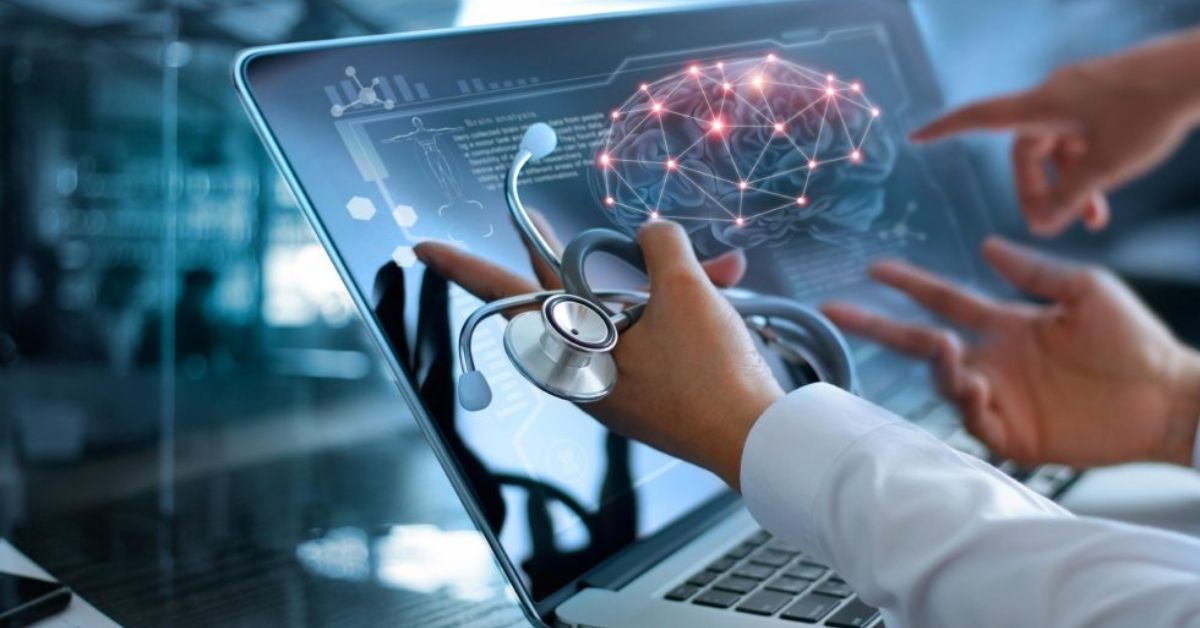
Using AI to Predict Complications Before and After Surgery
Although surgery has grown in popularity among surgeons, it is associated with a complication risk of up to 30%. This is also true for robot-assisted and minimally invasive surgeries such as Robotic Partial Nephrectomy. The morbidity of partial nephrectomy is caused by a mix of tumor complexity, patient-related complexity, tumor surroundings, and surgeon-related complications.
However, when accurate Machine Learning (ML) models are used in clinical practice, they can play a key role in predicting adverse events and improving patient outcomes. Narrow-focused AI models can be utilized to estimate the likelihood of intraoperative (during surgery) and post-operative (after surgery) events associated with a patient.
How is this Beneficial?
With the application of ML models to surgery and establishment and implementation of situation-specific intervention procedures, the surgeon can take advantage of this window of opportunity to manage patient risks. Predictive models developed in this manner can assist surgeons in identifying high-risk groups, anticipating significant occurrences, and planning treatment measures to enhance patient outcomes. A great case of this in action was performed by RediMinds.
RediMinds worked with the Vattikuti Foundation to create models for predicting intraoperative complications, or in other words, complications before and after surgery, and 30-day morbidity. The models were built using a multi-institutional dataset that was focused on a specific cohort of kidney cancer patients who underwent Robotic Partial Nephrectomy.
Machine learning and AI models performed well in this study in predicting problems during surgery and 30-day complications following surgery. These tools could be applied to real life clinical settings, which would allow the centers to utilize the models to identify patients who are likely to experience a problem before or after surgery, as well as to innovate intervention methods and offer feedback on model performance. This would also allow researchers to track the models’ performance in a clinical context.
Model Development
Here’s a brief rundown of RediMinds’ model deployment process for this study. First, historical data from patients who underwent robot-assisted partial nephrectomy is obtained, including demographic, pre-operative, intra-operative, and follow-up information. The next step, the data engineering process, then includes pre-processing historical data and defining features that will allow machine-learning algorithms to be trained on the data.
Next, model training and model validation models are trained, and the model with the greatest performance on unseen test data is chosen. The model with the highest performance is put in a clinical setting for further evaluation. The final step is the model monitoring, which is the process of comparing model predictions against ground truth to see if the model is operating as expected.
Final Thoughts
Surgeons strive to make their actions and decisions to treat patients under their care as predictable as possible. With the successful application of ML, it is possible to predict complications before and after surgery, which could be consequential to the patient’s surgical outcome.
This approach and methodology may be used in any surgical circumstance across the healthcare spectrum. It is not restricted to Robotic Partial Nephrectomy patients. With adequate data and continued research, the next generation of assistive AI surgical tools can be built utilizing the technique that RediMinds used.
To learn more, check out the case study
This isn’t the only way that our research into AI in healthcare is improving patient outcomes.
Check out our guide to 6 exciting applications of AI in healthcare.