Audio Deepfake Detection
The Challenge
While recent advancements in deepfake audio detection and countermeasure strategies show promise, it’s crucial to note that many of these solutions have been developed and tested using static audio recordings. These recordings typically last between 2 to 10 seconds and have limited variations in background noise, speaker count, artifacts, and recording conditions.
We refer to models trained on such static audio recordings as static deepfake audio models. However, these models may not consistently perform well in real-time scenarios, like continuous audio streams from applications on a device or communication platforms. For example, in a Teams group call, static deepfake models may struggle due to their lack of adaptability to dynamic variations in real-time conversational speech data.
This study systematically evaluates the viability of using static deepfake audio detection models in real-time and continuous conversational speech scenarios across communication platforms.
Exploration of Deepfake Creation Tools: Our journey began with an in-depth analysis of available deepfake tools, ranging from audio-specific platforms like Voice.ai, Eleven Labs, and Resemble AI, to video manipulation tools such as Avatarify and DeepFaceLive. This exploration provided us with a foundational understanding of the mechanisms behind deepfake generation.
02
Deepfake Generation for Testing: Leveraging virtual audio cables and Open Broadcaster Software, we created a diverse set of deepfake samples for testing. This step was critical in developing a detector that could identify a wide array of deepfake techniques.
Focusing on Audio Deepfakes: Given the prevalent use of audio deepfakes in scams, our detection efforts concentrated on audio analysis. We explored various deep learning methodologies, ultimately selecting a ResNet-based architecture for its superior performance in identifying fake voices.
Model Evaluation: Our evaluation framework utilized k-fold cross-validation and industry-standard metrics like EER and t-DCF. These methods ensured our models were rigorously tested for reliability and accuracy across diverse scenarios.
Application Development: To make our solution accessible, we developed an application for MAC, Windows, and Linux . This app allows for real-time audio detection from various sources, employing virtual audio cables for seamless integration.
06
Addressing Real-World Challenges: Our investigation revealed challenges in deploying static audio deepfake detection models on real-time communication platforms. The study suggests that future efforts should concentrate on developing efficient audio deepfake detection models capable of real-time prediction in such platforms.
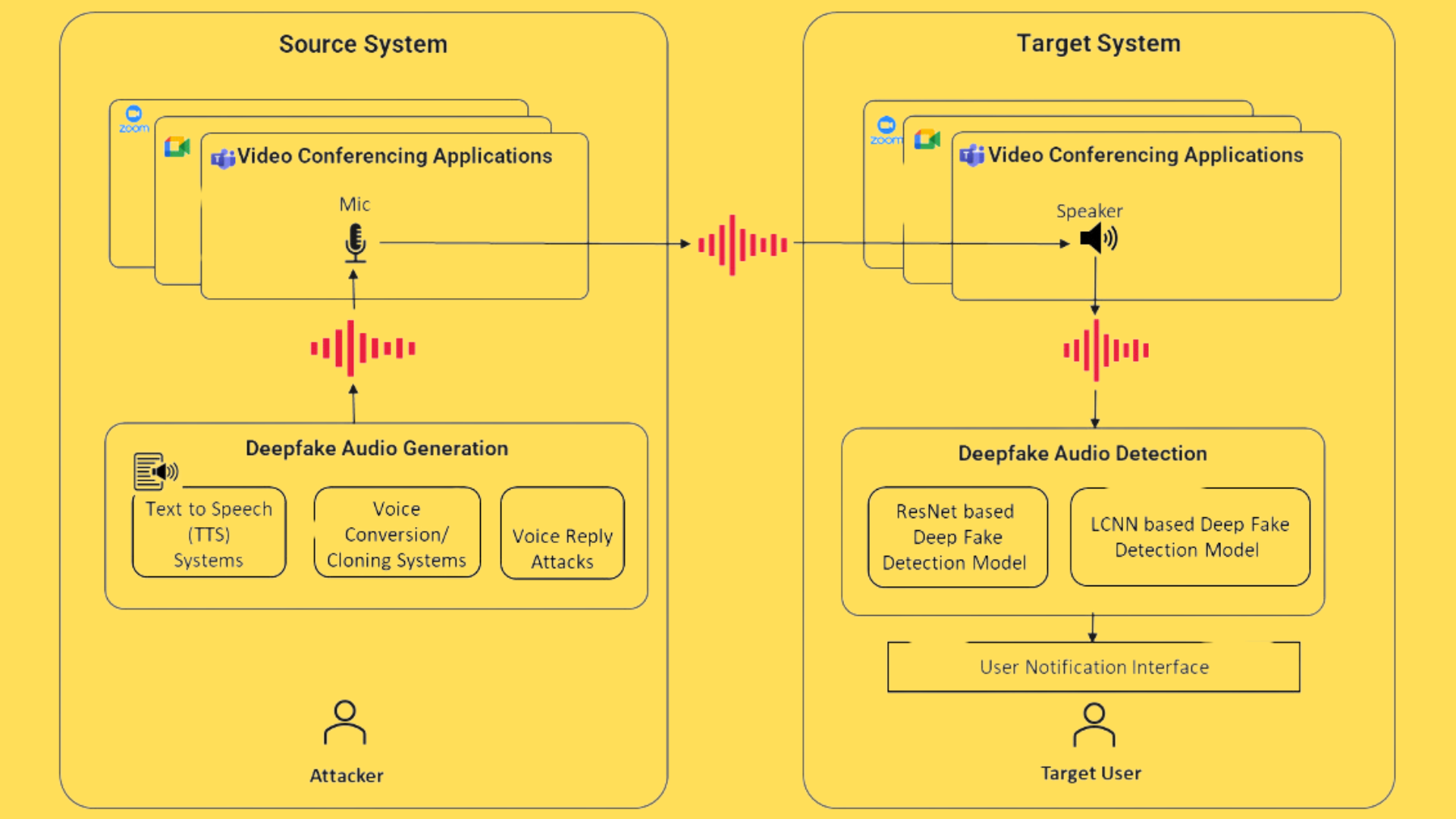
Results
Our audio deepfake models achieved notable results, with the leading model achieving an EER of 7.39 and a t-DCF score of 0.215 on the ASVspoof 2019 dataset, surpassing the baseline results of the ASVspoof 2019 challenge. However, when evaluated on real-time and continuous data using our Teams dataset, weaknesses in their performance were revealed.
Conclusion
The Audio Deepfake Detector project underscores RediMinds’ commitment to advancing AI in a manner that prioritizes security, privacy, and ethical considerations. Through a meticulous, step-by-step approach, we have created a cutting-edge tool that significantly enhances our ability to distinguish between real and manipulated audio. As we move forward, our focus remains on continuous improvement and adaptation to emerging threats, ensuring our solutions remain at the forefront of the fight against digital misinformation.
More about it
More details of our work can be found in our associated publication.